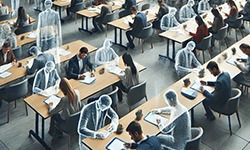
In the realm of research studies, you mostly test the impact of an independent variable on its dependent variable. However, if participants occasionally withdraw before a study’s completion, the link between those variables may not be proven correctly. This dropout, also termed as attrition, is not just a logistical challenge, it can also introduce a significant research bias. This article delves into the concept of attrition bias, how it is caused and how to prevent it.
Definition: Attrition Bias
Attrition bias, also called subject mortality, refers to the selective dropout of partakers in research studies. These dropouts may have unique characteristics that are important to the research subject. Therefore, their dropping out may result in a significant difference between the samples at the beginning and end of the study.
Printing Your Thesis With BachelorPrint
- High-quality bindings with customizable embossing
- 3D live preview to check your work before ordering
- Free express delivery
Configure your binding now!
Types of attrition bias
There are two types of attrition, which affect a study in different ways. Random attrition is, as the name says, a random dropout of participants, while attrition bias follows a systematic loss of responders.
- Random attrition is an error where the participants who drop out are comparable to those who stay. Eventually, it is simply a loss of participants. While it may not directly skew the results of your study, you are still losing statistical power since your whole sample is shrinking.
- Attrition bias, on the other hand, is systematic. This means that the dropouts have different characteristics than the ones who stay in the study. Even a few dropouts can have an impact on the results.
Causes
There can be many reasons behind attrition bias that are often not random but correlate directly with the study design. Some key reasons for attrition bias are listed below.
- Duration: Long studies are more prone to attrition because the participants may lose interest or need in the research. This can lead to them dropping out before the study is done.
- Effort: If the study demands a lot of effort from its participants, they are more likely to drop out. Factors could be the time they have to invest into answering questions or even physical activity in case of a sports related study.
- Demography: Age, health or social status can also influence dropout rates due to a different set of priorities in life or a change in them.
- Rewards: Participants usually expect some kind of reward for participating, whether this is something material or even knowledge. If they do not feel like they gain anything from participating, they are more likely to drop out of the study.
- Adverse events: Especially in clinical studies, there can be unwanted side effects to the tested drugs, but other kinds of research may also have adverse events that lead participants to drop out.
Problems
While some attrition is always expected in research, when it turns systematic, that is when problems start to show themselves. The effects of attrition bias can be both internal and external validity of the study.
- Internal validity: When participants with the same characteristics drop out of the study, this can lead to an over- or understatement of the results.
- External validity: Having dropouts with similar characteristics can result in an underrepresentation of that group in your study. Your sample does not represent the entire population anymore, and thus the research loses its validity due to attrition bias.
Detect attrition bias
Even with the tips above, you cannot eliminate attrition 100%. The key to detecting attrition bias is to compare the participants who stay in the study and those who drop out. You can use baseline data, like demographic variables (age, gender, ethnicity, education, etc.) and other variables of interest to compare the participants that leave and stay.
Sometimes attrition bias may not be so easy to detect. This is why it is important to question the dropouts and explore their reasons for leaving the study to spot possible hidden attrition bias.
Examples |
Age |
Ethnicity |
Gender |
Level of education |
Socioeconomic status |
Treatment or control group assignment |
Level of dependence |
Account for attrition bias
After detecting attrition bias, it is important to know how to continue the research. There are some ways to still continue the study, even if it may be biased. Some possible measurements will be explained in the following.
- Transparent reporting: If you recognize attrition in your study, it is essential to be open about it when showing the results. To keep credibility, you need to admit where and how bias affected your research and what methods you used to account for it.
- Sensitivity analysis: Furthermore, you can create different scenarios to follow up on dropout reasons. Then you examine what effect each scenario has on the results of the study. This allows you to draw conclusions about the actual dropouts and be more precise in questioning them.
- Multiple imputation: In this case, you replace the missing data with observations and expectations, imitating the outcome of the study without dropouts.
- Sample weight: Another method to account for attrition bias is to give the answers of participants, which are similar to the dropouts, more weight. This way you can try to make up for the missing samples while approximating the original distribution.
Prevention
There are a few rather simple ways to prevent attrition bias while designing the study. The key point is to make your study appealing and easy to handle for the participants. A few preventive measures to keep attrition rates low are listed below.
- Oversampling: Sample more participants than you actually need to equal possible attrition out.
- Communication: Keep your participants engaged, send them reminders for follow-up surveys but try not to overdo it. Otherwise, they might become annoyed with the study.
- Convenience: Make sure your surveys are convenient for the participants. Set deadlines long enough to not pressure them, keep the questions and the whole survey short and quick to handle.
- Compensation: Lure your participants with rewards after completing the surveys. These can be either monetary or non-monetary, depending on the interests of your participants.
- Participant information: Collect detailed information about your participants, firstly to look for similarities among dropouts, but also to keep in touch with them if they are going through major changes in their lives that should be noted.
FAQs
Attrition is when participants in research studies drop out mid-research, causing significant impacts on the outcome.
Another name for attrition is subject mortality. While many people think that subject mortality is when participants die, its true definition is when participants leave a study before it ends.
Attrition bias can be reduced by compensating participants, keeping the surveys as convenient as possible and communicate with the participants to keep them engaged in the study.
Attrition bias can distort the findings of your research. For instance, it can result in inaccurate findings due to the differences in the initial and end sample. It also affects internal and external validity, leading to over- or understated results.
To account for attrition bias, it is important to stay transparent when presenting your results, admitting if your study has been biased. Furthermore, you can change the sample weight or simulate the missing data with the help of statistics or your own observations.