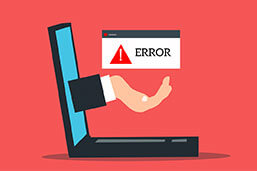
Understanding the distinction between random and systematic errors is crucial in academic writing, particularly in research methodology. Random errors occur due to unpredictable factors and can affect the accuracy and precision of measurements or data. On the other hand, systematic errors arise from consistent flaws in the measurement or data collection process, highlighting the importance of meticulous methodology to minimize bias and ensure reliable research outcomes.
Definition: Random vs. systematic errors
In random vs. systematic errors, the former (random) is a chance difference between observed and true values. By chance, it means that they are not intended. For instance, a random error may result from a researcher misreading the weighing scale.
In random vs. systematic errors, the latter (systematic) is a consistent difference between observed and true values. This error is usually consistent. For example, a systematic error occurs when a miscalibrated scale may register inaccurate weights.
numerous advantages for Canadian students:
- ✓ 3D live preview of your configuration
- ✓ Free express delivery for every order
- ✓ High-quality bindings with individual embossing
Random vs. systematic errors
When comparing random vs. systematic errors, the latter is considered worse. In scientific research, random errors are not considered a mistake but a result of unavoidable variability in measurement. For instance, if you measure the same thing severally, you may get different results due to environmental factors and inaccurate instruments. However, measurement variability can affect your ability to conclude the relationships between the variables. Unfortunately, this issue is more common with systematic errors.
Let’s look at other differentiating elements of random vs. systematic errors.
Precision vs. accuracy: Random vs. systematic errors
Random errors primarily affect precision. Note that precision is how reproducible the same value is under equivalent conditions. In contrast, systematic errors affect accuracy, which is how close observed measurements are to the factual values.
For high precision, you need to get repeated observations as close to each other as possible. In contrast, for accuracy, you need to get the measurements in your observation as close to the targeted value (true) as possible.
Random errors introduce inconsistency between diverse measurements of the same things, while systematic errors tilt your measurements from the true value in a definite course.
For example, if you measure the same thing repeatedly, your measurements will vary around the true value when you only have a random error. Therefore, random errors are not so problematic, especially in studies with large samples.
In contrast, systematic errors are more of a problem because they can tilt your data, resulting in false or inaccurate conclusions. Eventually, you may obtain a false negative or positive deduction of the correlation between variables in your study.
Random error: Random vs. systematic errors
The impact of random error in your measurements is usually unpredictable. For example, the measurements may be higher or lower than the true score.
Random error is also known as noise because it blurs the factual score (true value) of what you want to measure.
How does a random error occur?
Random may occur from many sources, including:
- Natural disparities in the real-life or experimental context
- Using faulty instruments
- Differences between the participants or units in the study
- Poorly conditioned trial processes
Decreasing random error: Random vs. systematic errors
Random vs. systematic errors are always present in research. While you cannot eradicate random error 100%, the following methods can decrease it.
Measure multiple times
You can take the measurements repeatedly and then use their average as the observation value. Doing this will bring you closer to the factual score.
Using a larger sample
Increasing the sample size also helps reduce random errors because they cancel each other in different directions. Therefore, a larger sample will increase precision.
Control the variables
Conducting controlled experiments can also eliminate extraneous variables that cause random errors. However, controlled conditions must be present for all participants.
Systematic error
In random vs. systematic errors, the latter means that your measurements of the same thing will vary predictably. Therefore, every observed value will vary from the true score in the same course. For instance, they may all be less than the true value or more. In some cases, the variations may also feature the same amount.
Systematic error is also known as a bias because the values are skewed consistently, hiding the true score. Therefore, it may cause inaccurate deductions.
Two types of systematic errors
A difference between random vs. systematic errors is that systematic errors come in two quantifiable types. They are:
1. Offset/additive/zero-setting error:
- This occurs when the measurement scare is not calibrated to a correct zero point.
2. Scale factor/correlational systematic/multiplier error:
- This is when the values consistently differ from the factual score proportionally (like by 10%)
How does a systematic error occur?
Various things may cause systematic errors in research, like materials and data collection procedures. However, the most common (but not all) are:
Decreasing systematic error
The methods below can help you reduce systematic errors.
Triangulation
You use multiple techniques to record annotations instead of one instrument or method. For example, you can use a ruler and a string to measure participants’ wrist circumference instead of a tape measure only.
Regular calibration
Regular instrument calibration can help you maintain accuracy. However, it would help if you had an accurate reference to ensure proper calibration. You can also calibrate the observers or researchers by providing a standardized procedure for data recording and conducting routine checks.
Randomization
Random sampling can help prevent sample bias, a source of systematic errors. Additionally, using the random assignment technique to place participants in various treatment conditions can help.
Masking
Finally, you can hide the condition assignment from participants and researchers to get authentic observations without influence from experimenter expectancies and environmental demand characteristics.
FAQs
Random error is a coincidental difference between observed and factual values, while, systematic errors are proportional or constant differences between factual and observed values.
In random vs. systematic errors, systematic ones are worse than random errors. This is because random errors are usually a natural part of the measurement and have variability, while systematic ones may easily go undetected.
Random vs. systematic errors are both measurement errors. A measurement error is a difference between the values you observe during an experiment and the factual ones.
Yes, you can reduce the impact of random vs. systematic errors in scientific research by recognizing their source. Identifying whether they are random vs. systematic errors also helps because random vs. systematic errors have different reduction methods.