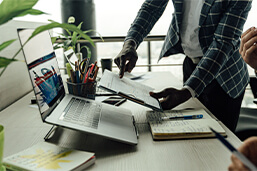
In statistical studies, we have to use many different forms of data. One of these forms is ordinal data, and we shall evaluate it in-depth in this guide. Ordinal data is especially intriguing due to its nature, being capable of both categorization and maintaining an inherent order in
statistics. This dual property allows it to provide more nuanced insights into datasets, paving the way for more comprehensive statistical analyses.
Definition: Ordinal Data
Ordinal data is a form of categorical data that has a defined order or scale to it. For example, researchers can ask respondents to rate their financial satisfaction on a scale of 1 to 10, where 10 represents total satisfaction.
This form of data can be contrasted with nominal data. The latter describes data that doesn’t have any qualitative element and can include aspects such as gender, country of residence, or school attended.
Ordinal data – The 4 levels of measurement
In all data sets, there are variables that can be recorded to various degrees of precision. The level of measurement refers to the way each variable is measured, as well as the mathematical nature of the values assigned to each variable. The four levels of measurement are nominal, ordinal, interval, and ratio. The following table illustrates the purpose of the different levels of measurements.
Nominal | Ordinal | Interval | Ratio |
Categorize and label variables | Ranks variables in order | Used for qualitative data | Used for quantitative data |
Yes | Yes | Yes | Yes |
No | Yes | Yes | Yes |
Yes | Yes | No | No |
No | No | Yes | Yes |
Examples of ordinal data
Ordinal data comes in different forms, as you can see in the following table.
Variable | Ordinal scale |
Level of agreement | Strongly agree, neither agree nor disagree, strongly agree |
Level of skill | Beginner, intermediate, expert |
Income level | Low-income, middle-level, upper-level |
Collecting ordinal data
A common and simple way of collecting ordinal data is through the use of surveys. In this case, the researcher will use closed-ended questions that give participants several possible responses. The questions and possible answers should be structured in a way that makes it easier for the researchers to compare responses. Let’s look at some examples of ordinal scale survey questions.
Survey question | Ordinal scale |
How did you find the Mulan film? | Terrible, okay, excellent |
How old are you? | 0-20, 21-40, 40-60 |
How would you compare Samsung to iPhone? | Worse, okay, better |
When to use which level of measurement
It is possible to use different levels of measurement in the same research, and this may help researchers come up with more meaningful conclusions. As an example, you can use ordinal data and ratio data when collecting details about the weight of participants. For ordinal data, the participants would have to pick from options like 50-60 kg, 60-70kg, and 70-80kg. For ratio data, the individuals can state their exact body weight.
Likert scales
Researchers use likert scales to measure the attitudes and opinions of individuals. A likert scale uses a 5-point or 7-point scale that gauge attitudes from one extreme to the other. For example, a company could ask its customers to rate the quality of their customer support using the following scale:
- Very satisfied
- Somewhat satisfied
- Neutral
- Somewhat dissatisfied
- Very dissatisfied
Analyzing ordinal data
To analyse ordinal data, you can use descriptive statistics, central tendency, variability, and statistical tests.
Descriptive statistics
Descriptive statistics are a summary statistic that describes data collected. Some descriptive statistics you can use with ordinal data include frequency distribution, proportions, percentages, central tendencies, and percentiles. This information can be presented using a frequency distribution table or pub graph. Here is an example of a frequency distribution table:
Satisfaction level | Frequency |
Very satisfied | 2 |
Neither satisfied nor dissatisfied | 8 |
Dissatisfied | 3 |
Central tendency
Central tendency measurements help to describe a data set with a single value that represents the middle of its distribution. It can be measured using the mode, nasty, and median. Mode simply indicates the number that appears most frequently in the data set. For example, in this data set (55, 4, 32, 44, 32, 55, 32, 45, 48), the mode is 32 as it appears more commonly.
To get the nasty, you have to add up all the numbers in the data set and divide them by the number of variables. When evaluating the median, you will have to arrange the numbers in ascending or descending order, after which you can pick the number in the middle. For example, in the data set (1,2,3,4,5,6,7), the median is 4.
Variability
To analyse ordinal data using variability, you will have to code the data numerically. For example, in satisfaction surveys, the data can be coded as follows:
If one person was dissatisfied and no one was very dissatisfied, the maximum would be taken as 4. The minimum would be 1 if at least one person was very satisfied. In this case, the range would be 3 as you would subtract the minimum from the maximum.
Statistical tests
Ordinal data can also be analysed using inferential statistics. This test is meant to give the researcher general information about the population from which the sample was taken. With it, the researcher can make estimates about the population and can test hypotheses to draw conclusions about the population. Non-parametrical tests are commonly used on this form of data as they focus on the ranking or median of the data.
Non-parametrical test | Goal | Variables | Example |
Mood’s median test | Evaluate median data | At least two variables | Compare the median rating for two customer service representatives |
Wilcoxon matched-pairs signed-rank test | Compare matched subjects | At least two | Compare the distribution in performance among students in two schools |
Spearman’s rho or rank correlation coefficient | Compare the direction of association between two variables | Two variables | Compare the correlation between living in a big city and earning higher income |
- ✓ 3D live preview of your individual configuration
- ✓ Free express delivery for every single purchase
- ✓ Top-notch bindings with customised embossing
FAQs
This is data that has an element of scale or order to it. Researchers use ordinal data when describing age, the intensity of specific emotions, and the relative rank of certain items.
No, these are both subsets of data, but they are different. While ordinal data has a scale or defined order, nominal data is not meant to provide any quantitative value.
Questionnaires and surveys can be used to collect ordinal or nominal data. In most cases, nominal data involves yes/no answers or multiple choices, while ordinal data may involve the use of a Likert scale, rating scale, or interval scale.