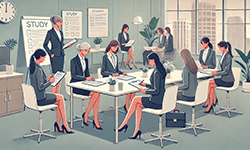
In the methodology of academic research, understanding and avoiding research bias is pivotal to ensuring the validity and reliability of your findings. Research bias can influence your study before, during and even after the trial, causing your study to lose representativeness. One type of research bias that happens before the trial is sampling bias, which will be introduced in the following article.
Definition: Sampling bias
Sampling bias is a type of research bias, where the selected participants do not represent the whole population or the whole target group of your study. This issue can make it harder to generalize the findings of the research, as it presents a threat to the validity and representativeness.
Types of sampling bias
There are a few different types of sampling bias, which can affect your research.
Probability sampling
Probability sampling is a method where all samples are chosen randomly. This lowers the risk of sampling bias, as no one can influence who gets chosen. There are, however, still a few types of bias that can still happen even with probability sampling.
- Simple random sampling bias occurs when the technique of random sampling, for example drawing lots or a random number dynamo, is flawed. This can happen due to technical inaccuracies, leading to over- or underrepresentation of certain groups.
- Systematic sampling is a method where you choose samples by a system, for example in certain distances on a list. If those happen to be not representative for the whole group, it still ends up as a bias, even though unintended.
- Stratified sampling divides the population into different subgroups, from which the samples are selected. These groups can be chosen by age, gender, family background, etc. However, if those subgroups are separated poorly or certain groups are not represented, stratified sampling bias happens.
- Cluster sampling starts by dividing the population into smaller groups or clusters before sampling participants from those randomly. If those clusters are not completely random or the selected participants still do not represent the entire population, the study results will be biased nevertheless.
Non-probability sampling
In non-probability sampling, the participants are not chosen randomly, which can easily lead to bias. Even though sometimes it can be necessary to not sample your participants completely random, there are quite a few biases you need to be wary of.
- Convenience sampling bias occurs when you sample your participants depending on availability or willingness to participate, as well as proximity, for example your friends. Those groups of people, however, often do not represent the population adequately and thus lead to biased results.
- In judgmental sampling, the researcher chooses their samples themselves, based on non-random criteria they deem relevant. This brings a certain subjectivity into the study, that can lead to them subconsciously sampling participants that seem to prove their hypothesis of research or simply do not represent the population as a whole.
- Quota sampling selects participants along certain quotas, for example age, gender or financial status. This should happen to make sure the demographic characteristics of the population gets represented. If the factors for the quotas are not chosen randomly or in a representative way, this can still lead to bias.
- Purposive sampling refers to a situation where the researcher purposefully selects certain criteria and samples participants according to these. While these criteria are chosen to match the objective of the research, if the samples are not representative of the population, the study will still end up biased.
Causes
Often, sampling bias results from other types of bias that influence the process of selecting participants. The most common causes for sampling bias are:
- Volunteer bias, where you sample participants that volunteer to join. These people may not represent the population as a whole because participating by choice is not something every type of person will do.
- Survivorship bias, where only successful people are sampled for the study. These people all have passed a certain trial, for example if you select your participants among university graduates, they all have “survived” university.
- Non-response bias, where some of your selected participants did not answer to your study for similar reasons. An example of this could be conducting a phone survey, where you call your samples along the day. However, people who are working at this time of the day will not be able to take the call, and thus they are left out in your research.
Impact
The consequences of sampling bias will most likely highly affect the validity and reliability of your study in many different ways.
- Overestimation or underestimation: Distorted results can lead to a wrong impression and thus wrong estimation.
- False Associations: Biased samples can also cause wrong associations between different variables that are actually not related, or you miss a connection that is actually existing but is not represented in your samples.
- Inaccurate conclusions: Wrong conclusions based on sampling bias can eventuatein wrong decisions and interventions resulting from the research.
- Limited applicability: A biased study does not produce reliable results, which means that it can not or just very limited used in research.
- Wasted resources: If your study is biased, you might need to redo the whole procedure, which means that you have to spend time and money again.
- Ethical considerations: Your participants might feel treated unjustly, especially if the biased results get published and thus misrepresent the population. Furthermore, the study might also encourage stereotypes or discrimination.
Detect sampling bias
As the impact of sampling biased studies can be quite intense for your research, it is important to know how to detect sampling bias in your findings. This can happen through data analysis, visualization or review of the data.
- Data analysis: During data analysis, make sure to compare your findings to existing research and statistics. Moreover, be wary if your own survey seems to prove your hypothesis perfectly because then it is highly likely that you experienced sampling bias.
- Visualization: Another method to check for sampling bias can be the visualization of your results through diagrams, heatmaps or comparative visualization. The visualized results may help you recognise possible flaws in your study design and sampling, as well as the results of your research.
- Review: The input from an independent expert or even your family and friends can help identify biases in your study. Furthermore, you can also conduct smaller pre-testing or pilot studies to double-check your results.
Avoid sampling bias
To make sure your study is valid and representative, it is essential to avoid sampling bias. The following tips might help you in conducting your research by limiting the impact of bias in your study.
- Stratified or random samples might limit the influence of bias in selecting your participants, while making sure that your group of participants still represents the population as a whole.
- Avoid convenience sampling as much as possible. Even though it might be easier to find accessible targets, they often do not represent the population enough to make your study valid.
- Follow up with those participants, that did not respond to your questions or calls. This way, you make sure every person you want to participate in the study actually does so.
- Oversampling is an easy way to limit the influence of sampling bias. If you sample more participants than are actually needed in your study, you increase the chance that no group is underrepresented.
FAQs
Sampling bias happens when the selected participants for a study do not represent the entire population, which leads to not representative results of the research.
A researcher can eliminate sampling bias by sampling the participants randomly, so every potential respondent gets an equal chance of taking part in the survey.
Bias poses a threat to external validity since the findings will be generalized to the population. It can lead to an overestimation or underestimation of the corresponding parameters in the population, wrong conclusions that lead to inappropriate decisions, which are taken according to the results.
If the sample bias is introduced by the wrong sampling method used by the researcher, the sample size will not reduce the bias. However, especially in random sampling, the probability of representing the population correctly, an increase in sample size does help to reduce bias.